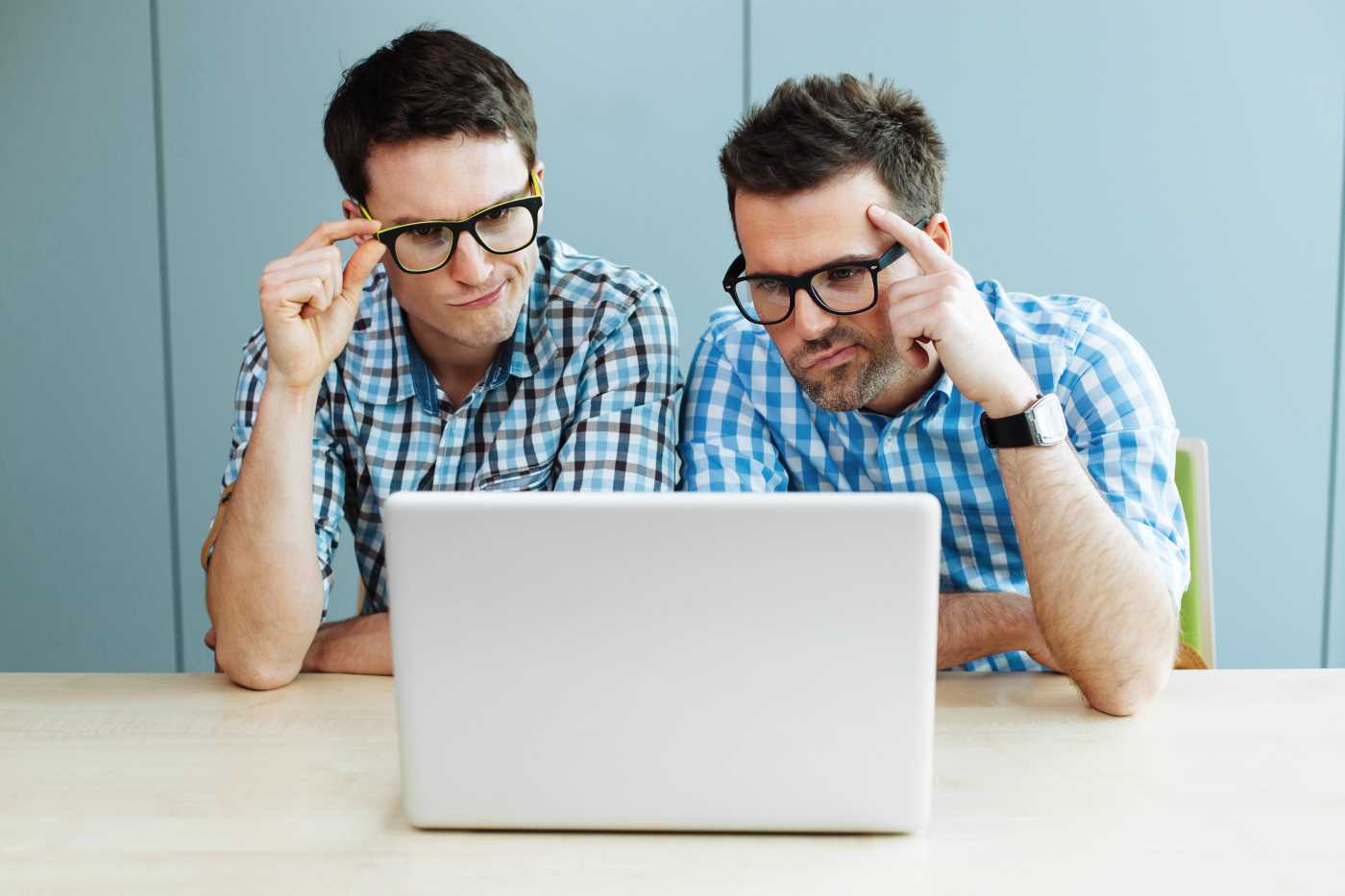
Do you want to get the best from social media monitoring? Or are you looking for the best social media monitoring tool to forecast your consumer behaviour during this global pandemic? Here are some important points before you make your decision.
There are approximately more than two hundred social media monitoring tools or software out there in the market.
The role of technology as a key enabler has transformed industries, marketers and corporate communications by gaining competitive advantage. Insights from social media monitoring are used to improve customer experience, increase brand loyalty and reduce reputation risks.
Social Media Monitoring Providers
If you are out there seeking for social media monitoring partners for your organization, there are two categories of social media monitoring providers that you should know:
- Tool-Based – software system providers for you to subscribe and perform in-house monitoring
- Service-Based – service providers that analyze your data and take care of everything you need from software licenses to data cleaning/processing.
Social Media Monitoring Processes

One of the underlying principles of the best social media monitoring tool or service provider lies in its ability to process data and information into actionable insights.
Actionable insights are defined when you are able to perform some level of action based on the information presented. Actions can include but not limited to defining a new strategy, focusing on areas that matter, realigning your campaigns, targeting your audience better or aligning your narrative and creatives.
However, how do we get the best from social media monitoring activities? Are tools the only answer or are there other approaches such as outsourcing social media monitoring to experts that can deliver better insights?
Here are top things that you need to know before subscribing to social media monitoring tools for your in-house needs, or outsourcing your social media monitoring activities to a team of experts.
Define your objectives clearly
Needless to say, the invention of social media monitoring tools was designed to assist businesses to automate part or most of their processes.
However, you need to define clear business objectives to extract maximum value from social media monitoring.
Here are some common social media monitoring objectives to choose from:
- To monitor reputation risks from negative sentiment from consumers about your brand
- To provide new insights to consumer behaviour or predict future consumer trends
- To improve customer experience through what been said on social media about your brands and others.
- To analyze competitors by identifying their marketing strategies
- To provide a baseline of your current brand – i.e brand health check and others, for further improvement
- To assess the effectiveness of your online or offline campaigns.
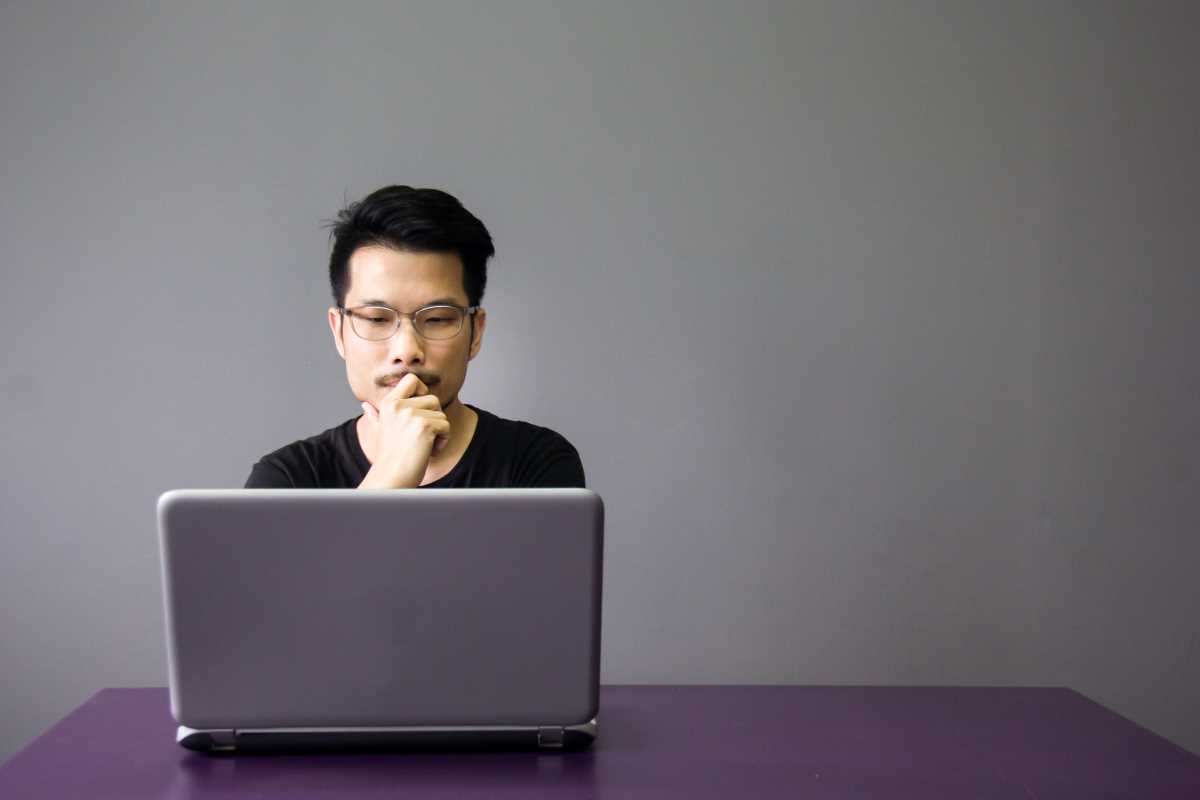
Tools bring efficiency in the expense of more resources
There are many organizations that view social media monitoring as an increasingly important part of the business. The exponential growth of social media data provides invaluable insights to communication managers and leaders wanting to be ahead of the innovation curve.
However, here is one thing that you need to know.
The efficiency of social media monitoring tools comes at an expense of more resources, additional capital expenditure and operating costs.
What used to be a one-person headcount to monitor social media activities – it is now has become a full-fledged unit with multiple skillsets to analyze meaningful social media data to achieve a set of business objectives.
Therefore, be prepared to invest in the right people with relevant skillsets.
Efficiency gain also means end users can enjoy a host of features and visualizations offered by social media monitoring tools.
However, there is a missing gap which remains unsolved by the tech industry until today:
How trustworthy are these insights? And are we seeing the right insights?
Automated tools have lower sentiment accuracy
Most social media monitoring tools have automated Machine Learning algorithm built-in into the system, however each of us today express ourselves differently in multiple languages.
Now here is a fun fact.
75% of the global internet users use their native language.
According to Statista, English represent 25% of the total language spoken on the internet and that includes in social media platforms. Chinese, Malay, Spanish and Arabic represent the remaining language used on the internet.
This brings a huge challenge for social media monitoring tools that rely on automated sentiment tagging.
Now, have you experienced a scenario below – i.e when sentiment was wrongly tagged by a social media monitoring tool? An example below was conducted for various topics for a large telco company in Indonesia using a social media monitoring tool.
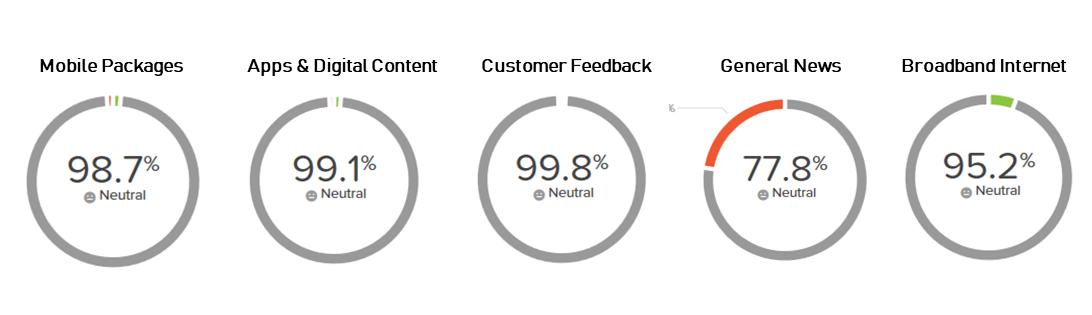
The result of automated sentiment analysis using a global social media monitoring tool. Analysis was performed on multiple topics (i.e domain) on a large telco company in Indonesia. Source: Berkshire Media Team analysis.
Now, researchers from the University of Zurich found that the sentiment accuracy in commercial automated social media monitoring tools (which processes text data from social media) is between 40% to 60%.
Sentiment accuracy from tools is between 30% to 60%
This bogs down to the complexity of understanding different languages based on keywords.
Understanding language remains a challenge
Language shapes the way we think and act. In markets where local and native language are intertwined with local dialects and slang, this brings unique challenges for automated social media monitoring tools.
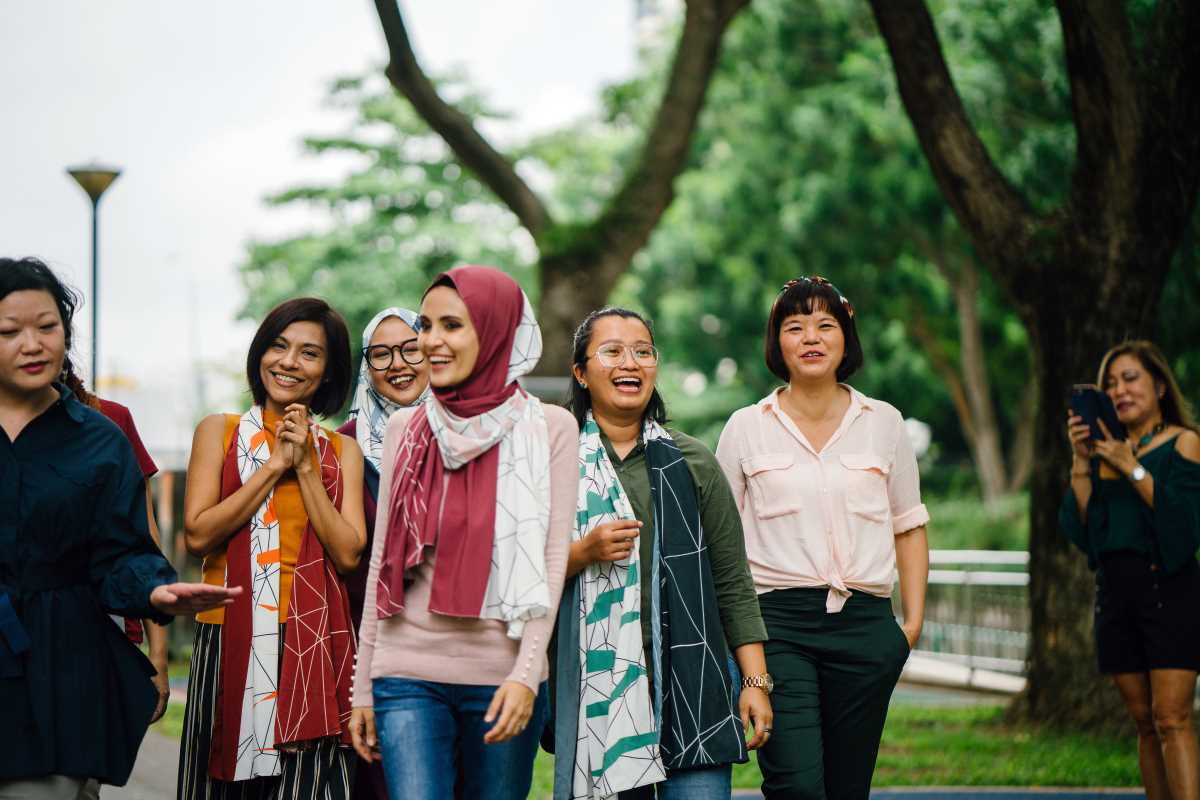
We found challenges faced by social media monitoring tools to analyze native languages in Asia. A good example is the Malay language which is widely spoken by several countries. Here is a quick background from Wikipedia:
Malay is an Austronesian language spoken in Brunei, Indonesia, Malaysia and Singapore, as well as parts of Thailand.
A language of the Malays, it is spoken by 290 million people across the Strait of Malacca, including the coasts of the Malay Peninsula of Malaysia and the eastern coast of Sumatra in Indonesia and has been established as a native language of part of western coastal Sarawak and West Kalimantan in Borneo.
It is also used as a trading language in the southern Philippines, including the southern parts of the Zamboanga Peninsula, the Sulu Archipelago and the southern predominantly Muslim-inhabited municipalities of Bataraza and Balabac in Palawan.
The Malay language is unique and filled with slangs and dialects across different regions – even within the same country.
Meanwhile, Indonesian Malay is significantly different from Malaysian Malay language. Even the words and slangs have different meanings.
The absence of dialects and slangs in languages have not been taken into account in social media monitoring tools today. Hence why we are witnessing more “neutral sentiment” each time results are presented by the tools.
This degree of error can be circumvented by a supervised machine-learning approach.
Clean data and machine learning text algorithms
Users are over dependent on automated approach using technology to process text data despite that the Artificial Intelligence (AI) powered algorithms are relatively at its infancy. We estimate that it takes another 10 years for the text algorithm to reach its maturity stage.
Having said that, with millions of unprocessed social media data, it takes a radical shift for the social media monitoring tools to process data into meaningful and actionable insights.
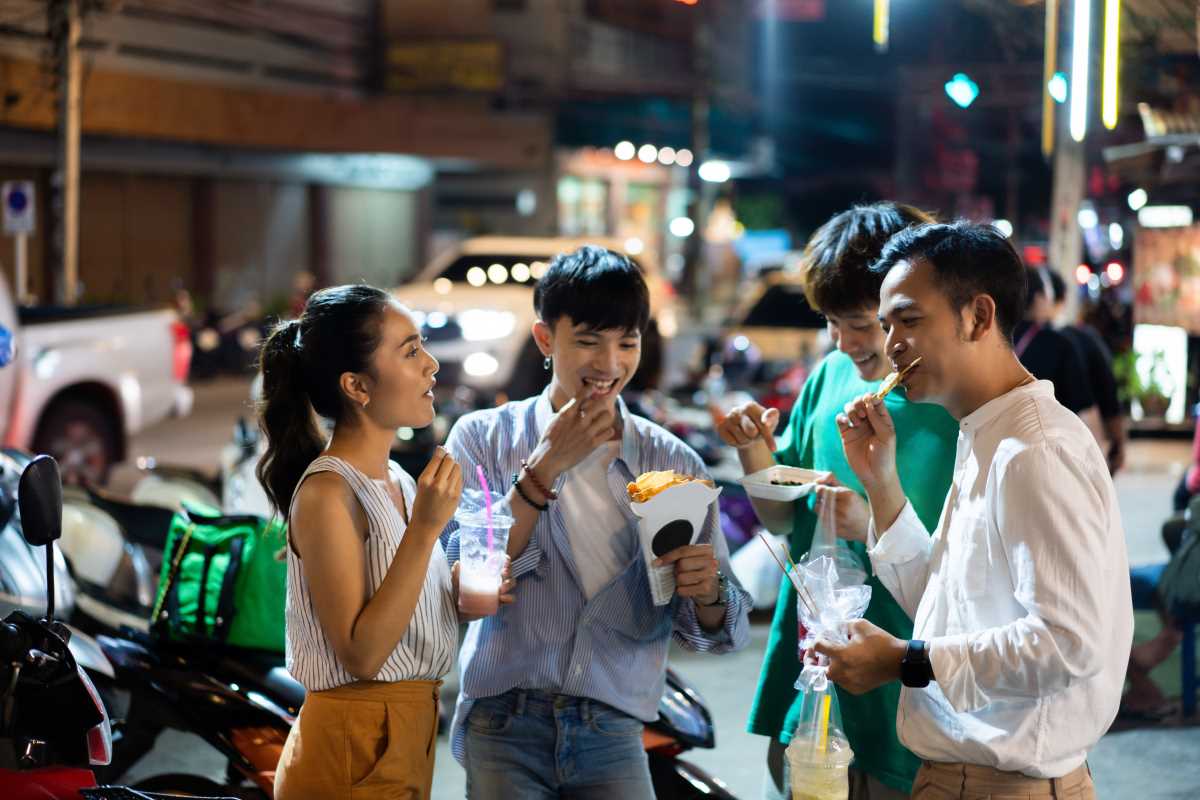
Existing machine learning algorithms that are built-in (and not customizable) by many social media monitoring tools out there can only mean one thing – high error rate and very low sentiment accuracy to monitor brands, topics and even issues. This can potentially lead to wrong decisions by campaign managers, business leaders and policy makers.
As language evolves with new keywords found each year, dialects and slangs can only be processed more accurately via a semi-supervised machine learning approach.
Below is an example of a semi-supervised machine learning approach using text algorithm SENTIROBO® to analyze sentiment from 1.68 million social media data during the COVID-19 pandemic using multiple languages, dialects and slangs.
Before Data Cleaning
& Sentiment Processing
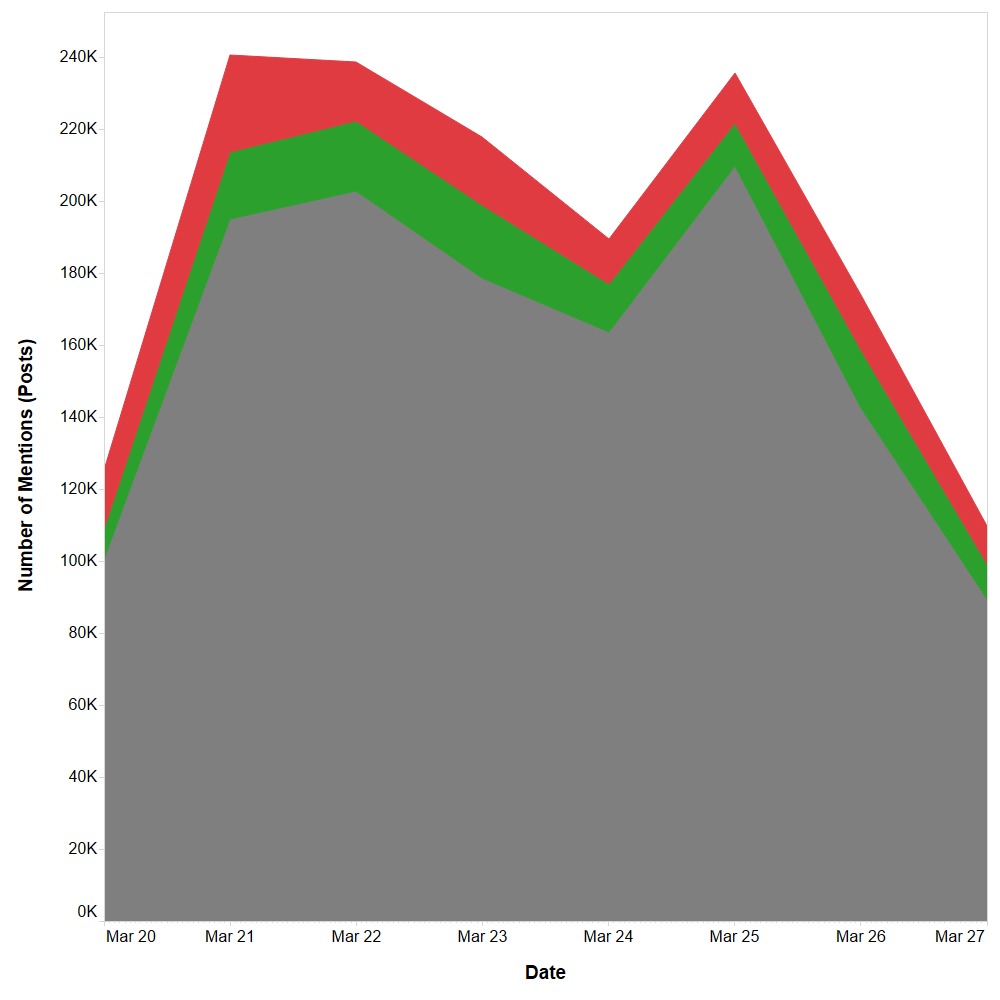
After Data Cleaning
& Sentiment Processing
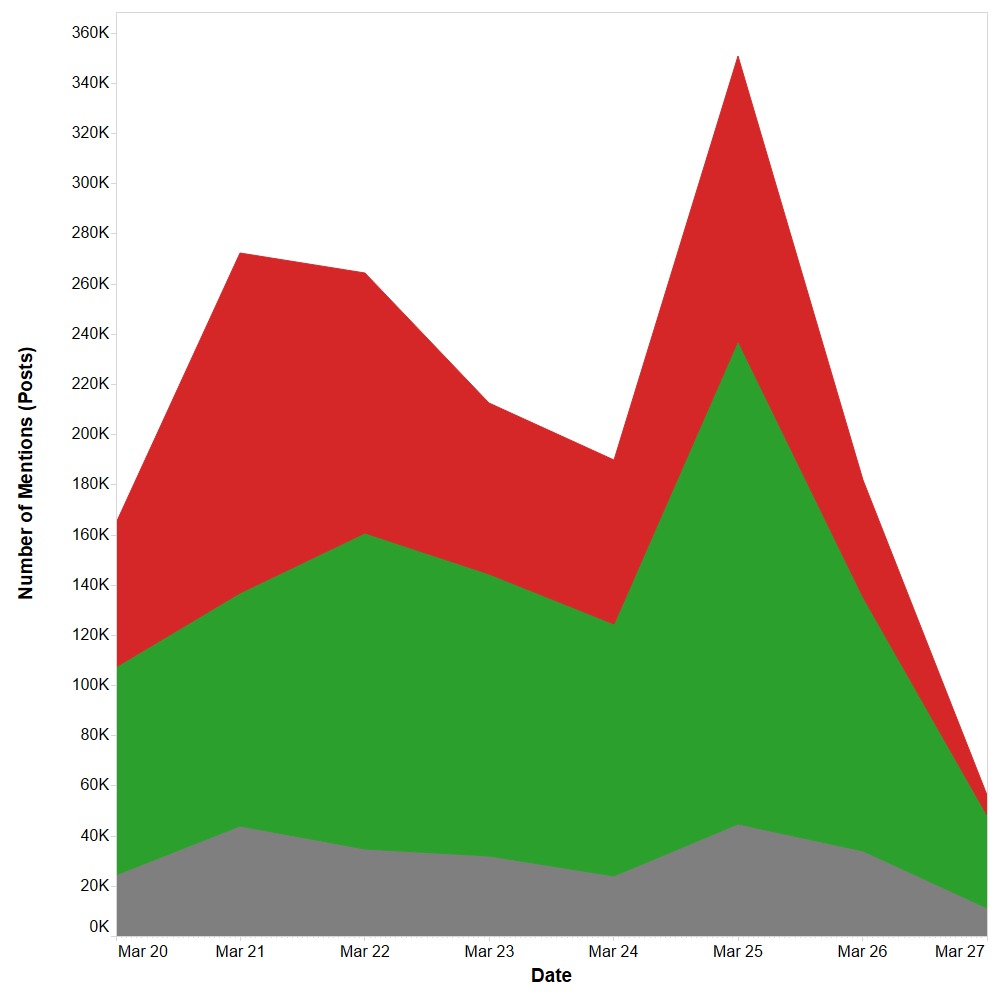
The above results showed before and after data cleaning and data processing with the inclusion of contextual keywords that reflect sentiment polarities that exists in social media posts. With this approach, we managed to successfully reduce the data error and increase the sentiment accuracy to an acceptable level of more than 70%.
In our past research studies, our bespoke approach has enabled sentiment accuracy to be measured by up to 79% and close to 100% in other case studies.
Closing Thoughts
Each social media monitoring tool has its own strengths and weaknesses. For end consumers, it means understanding various functionalities of a system or software in order to better serve the business needs.
Beyond vanity metrices and fancy data visualizations, social media monitoring is a growing industry with plenty of opportunities for end users and businesses to leverage to arrive at better insights.
If data tells a story, then the story should lead to actions. Beyond the conventional wisdom of AI, we ought to bring ourselves to this point:
Are we over dependent on technology to automate our social media monitoring tasks or do we treat technology as an enabler?
If you choose the latter, it means you need to consider getting the best People, Process and Technology to arrive at the best possible insights for your organization. That means equipping your internal team with the right knowledge to perform data mining, data cleaning, data processing and interpreting those data into meaningful insights.
In light of the global uncertainty – finding the need to balance between speedy business recovery, brand loyalty, and consumer intelligence requires a delicate approach.
Now, this leads to the final question: should you invest in an in-house social media monitoring system, or getting a social media monitoring agency to provide end-to-end services?
The merits of the answer to the above question are rather compelling, but we leave that to you to decide.
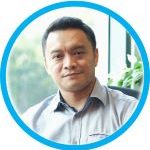
About the Author
Shahid Shayaa is the founder and managing director of Berkshire Media. He is on a mission to drive data-driven insights and communication strategies for companies. Specializing in machine learning text algorithms, he publishes various scientific papers on social media analytics to better understand consumer sentiment, emotions and behaviour for marketers and campaign managers.
References:
Mark Cieliebak, Oliver Dürr, Fatih Uzdilli (2013), Potential and Limitations of Commercial Sentiment Detection Tools. – ESSEM@ AI* IA