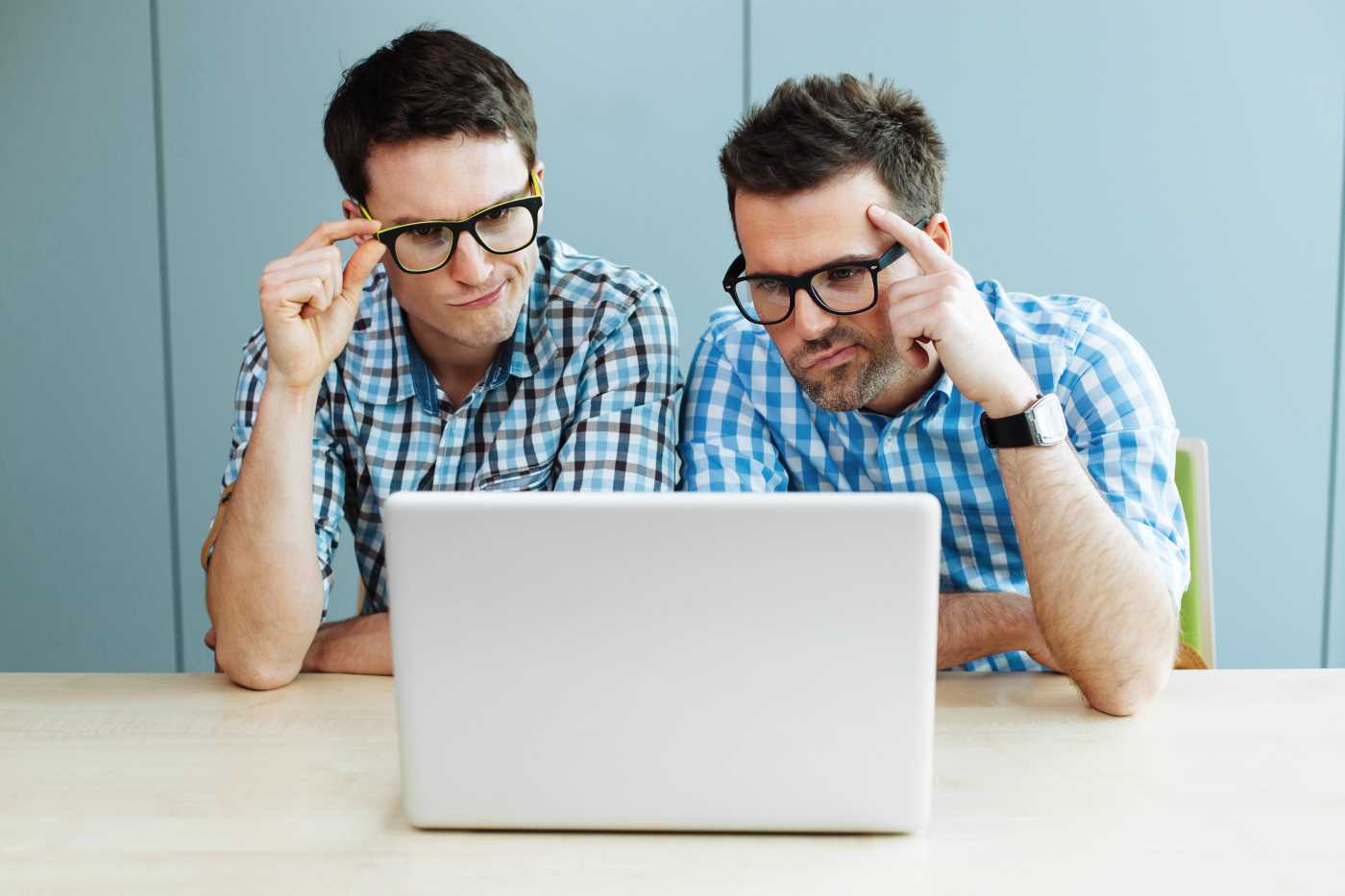
The short answer is, yes, social listening or monitoring tools enable you to predict issues or crisis before it happens. However, it requires a deep-dive analytics approach by applying layers of statistical methods such as linear or non-linear polynomial regression and other probabilistic modelling due to the stochastic nature of social media data.
And in this article, we shall share more insights how predictive analytics on social media data can be helpful in anticipating various types of communications and operational crisis.
Overview
Social listening tools often offer predictive analytics capabilities but more often than not, AI-driven or automated detection engine does not offer the full suite of statistical-based approach to predict a crisis before it happens.
“Social data signals are often categorized as stochastic and this random pattern makes it challenging for most automated social listening tools to predict future occurrence of a given topic or issue”, said Shahid Shayaa, CEO & Founder of Berkshire Media.
Social analytics companies such as Berkshire Media helps many Malaysian companies predict recurring issues that affects brand perception and reputation using a set of tools and methodologies.
According to communications and data scientists, you need to meet several conditions such as access to historical data to ensure you arrive at a better prediction. Using a set of statistical methods and leading indicators, this will result in measuring the probability of the issue being a crisis.
Predictive Analytics For Various Types of Crises
Here are types of crises that you can predict using social data or social insights:
1. Reputational Crisis
Definition: Negative sentiment surrounding a brand, person, or executive that affects public trust.
Examples:
- Viral backlash over insensitive ad campaigns.
- Accusations of greenwashing or unethical behavior.
- Executive misconduct or controversial statements.
How Social Data Helps:
- Early detection of negative sentiment spikes.
- Monitoring trending hashtags or keywords tied to scandals.
2. Product or Service Failure
Definition: Widespread complaints or defects reported by users that gain traction.
Examples:
- Reports of defective consumer products (e.g., battery explosions, spoiled food).
- Outages or errors in digital platforms or services.
How Social Data Helps:
- Identifies recurring complaints.
- Maps geographic hotspots or user segments affected.
3. Customer Service Crisis
Definition: Mass dissatisfaction due to poor customer support or response time.
Examples:
- Airline passengers live-tweeting delays or mistreatment.
- E-commerce delivery failures during peak seasons.
How Social Data Helps:
- Flags mentions with negative sentiment and support language (e.g., “no reply,” “waited 2 hours”).
4. Regulatory or Legal Crisis
Definition: Legal investigations, policy violations, or regulatory breaches that surface online.
Examples:
- Data privacy violations (e.g., GDPR non-compliance).
- Investigations into labor or environmental violations.
How Social Data Helps:
- Captures news breakouts and public reaction in real time.
- Detects whistleblowing or leaks before mainstream coverage.
5. Workforce/HR Crisis
Definition: Internal employee issues that become public and damage brand perception.
Examples:
- Layoffs and internal disputes aired on LinkedIn or Twitter.
- Workplace harassment allegations or labor strikes.
How Social Data Helps:
- Tracks employee sentiment on public platforms.
- Identifies viral employee posts or Glassdoor patterns.
5. Workforce/HR Crisis
Definition: Internal employee issues that become public and damage brand perception.
Examples:
- Layoffs and internal disputes aired on LinkedIn or Twitter.
- Workplace harassment allegations or labor strikes.
How Social Data Helps:
- Tracks employee sentiment on public platforms.
- Identifies viral employee posts or Glassdoor patterns.
6. Ethical or Social Justice Crisis
Definition: Public outrage tied to social, racial, gender, or environmental insensitivity.
Examples:
- Brands being called out for racism, sexism, or cultural appropriation.
- Ignoring major social movements (e.g., #MeToo, #BlackLivesMatter).
How Social Data Helps:
- Detects alignment/misalignment with public mood and events.
- Maps activist sentiment and influencer amplification.
7. Cybersecurity/Data Breach Crisis
Definition: Leakage of sensitive data or hacking incidents that spread quickly on social platforms.
Examples:
- Customer data leaks, account hijacks, ransomware attacks.
- Exposés of password vulnerabilities or phishing scams.
How Social Data Helps:
- Identifies early mentions by cybersecurity experts or affected users.
- Detects phishing pages or complaints across forums and Reddit.
8. Supply Chain or Operational Disruption
Definition: Delays or disruptions in product delivery or availability.
Examples:
- Shortage of goods (e.g., fuel, medical supplies, semiconductors).
- Warehouse issues or port delays.
How Social Data Helps:
- Captures real-time public frustration.
- Identifies geographic areas most vocal or affected.
9. Financial/Investor Confidence Crisis
Definition: Decline in stakeholder trust based on rumors, poor earnings, or executive exits.
Examples:
- Insider trading rumors, major resignations, or earnings misses.
- Public investor backlash on platforms like X (Twitter), Reddit, or StockTwits.
How Social Data Helps:
- Detects early panic signals in financial communities.
- Monitors stock sentiment and keyword frequency (e.g., “sell,” “losses”).
10. Geopolitical or Environmental Crisis
Definition: Natural disasters, conflict, or external events that impact operations or safety.
Examples:
- War zone disruptions, sanctions, or diplomatic fallout.
- Wildfires, floods, or hurricanes affecting business continuity.
How Social Data Helps:
- Real-time local updates from affected regions.
- Monitoring government or aid organization communications.
Predicting a crisis: What can we expect?
Since each social media data is unique and random, predicting whether the issue can occur in future requires applying regression modelling on a set of historical time-based series social data before calculating the probability of occurrence.
For you to have better prediction, you need to ensure that:
- You have a set of clean data extracted using relevant keywords pertaining to that topic
- You have access to historical (past data) in order to predict the future
- You have sufficient amount of data (volume of mentions) to predict the crisis
Here is an illustration when we apply various regression lines (linear, non-linear or polynomial) on social data for a given topic (or issue).
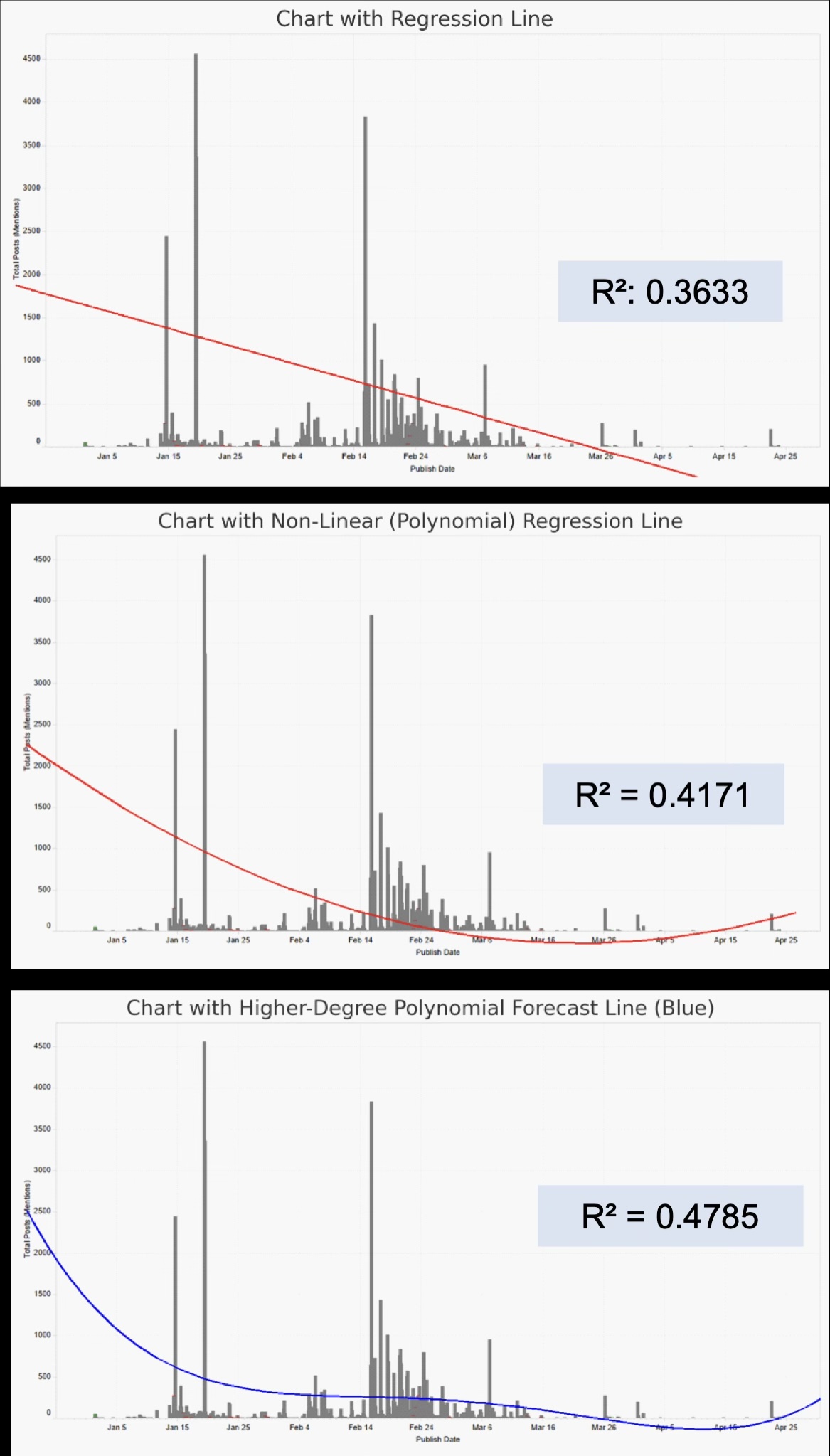
In the example above, higher degree polynomial forecast line brings better fit denoted by the higher R2 values. In this case, we calculate the probability of it recurring in future at 83%, and the regression model suggests a high likelihood of resurgence, given the historical pattern of spikes and the current trajectory shown.
Never fall for the hype. Automated social listening tools have less predictive capabilities in reality and only few companies are well equipped to predict recurring issues, trends or behaviour from large social media datasets.
While this example provides a basic illustration of what can be done to predict a crisis from social media data or listening tools, there are further steps and deep-dive analysis that may be required to understand abnormalities, user behaviour, recurring patterns and factors shaping the issues.
Parting Thoughts
The rise of automation that drives AI-enabled social listening and media monitoring tools may not offer the predictive analytics solution that one expects.
You cannot predict future risks without sufficient historical data.
In the realm of data science, predicting a crisis or consumer behaviour from social media is still largely handled by industry experts with experience in data processing, statistical analysis, text mining and sentiment tagging.
And for that reason, social analytics companies such as Berkshire Media are highly sought after by large organizations in Malaysia to harness more value from social data in the last decade.
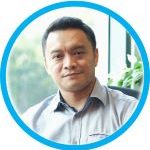
About the Author
Shahid Shayaa is the founder and managing director of Berkshire Media. He specializes in data-driven communication strategies and insights using social data analytics, social media monitoring tools and machine learning text algorithms for more than 13 years. As an expert in the field of media monitoring, issue management and managing reputation risks for companies, he is involved in various research studies in this field and published various scientific papers on social data analytics, sentiment analysis and back-end algorithms on consumer sentiment, emotions and behaviour for marketers and campaign managers.
His research work and studies have been cited more than 467 times, inspiring new research in the field of social analytics in Malaysia. You may view his work here.